RAFT: In-Domain Retrieval-Augmented Fine-Tuning for Language Models
Best AI papers explained - A podcast by Enoch H. Kang
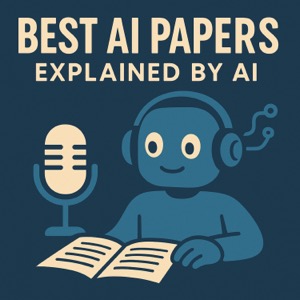
Categories:
This paper introduces Retrieval Augmented Fine Tuning (RAFT), a novel training method designed to improve large language models' ability to answer questions accurately within specific domains when provided with relevant documents. RAFT trains models to effectively utilize provided documents by incorporating both helpful and distracting information during fine-tuning, encouraging the model to discern and cite relevant passages. The research demonstrates that RAFT enhances performance on domain-specific question answering tasks across various datasets compared to standard fine-tuning approaches, even when using retrieval-augmented generation. Key elements of RAFT include training with distractor documents and generating chain-of-thought reasoning grounded in the provided context.